Automatic whole cell organelle segmentation in volumetric electron microscopy
Cells contain hundreds of different organelle and macromolecular assemblies intricately organized relative to each other to meet any cellular demands. Obtaining a complete understanding of their organization is challenging and requires nanometer-level, three-dimensional reconstruction of whole cells. Even then, the immense size of datasets and large number of structures to be characterized requires generalizable, automatic methods.
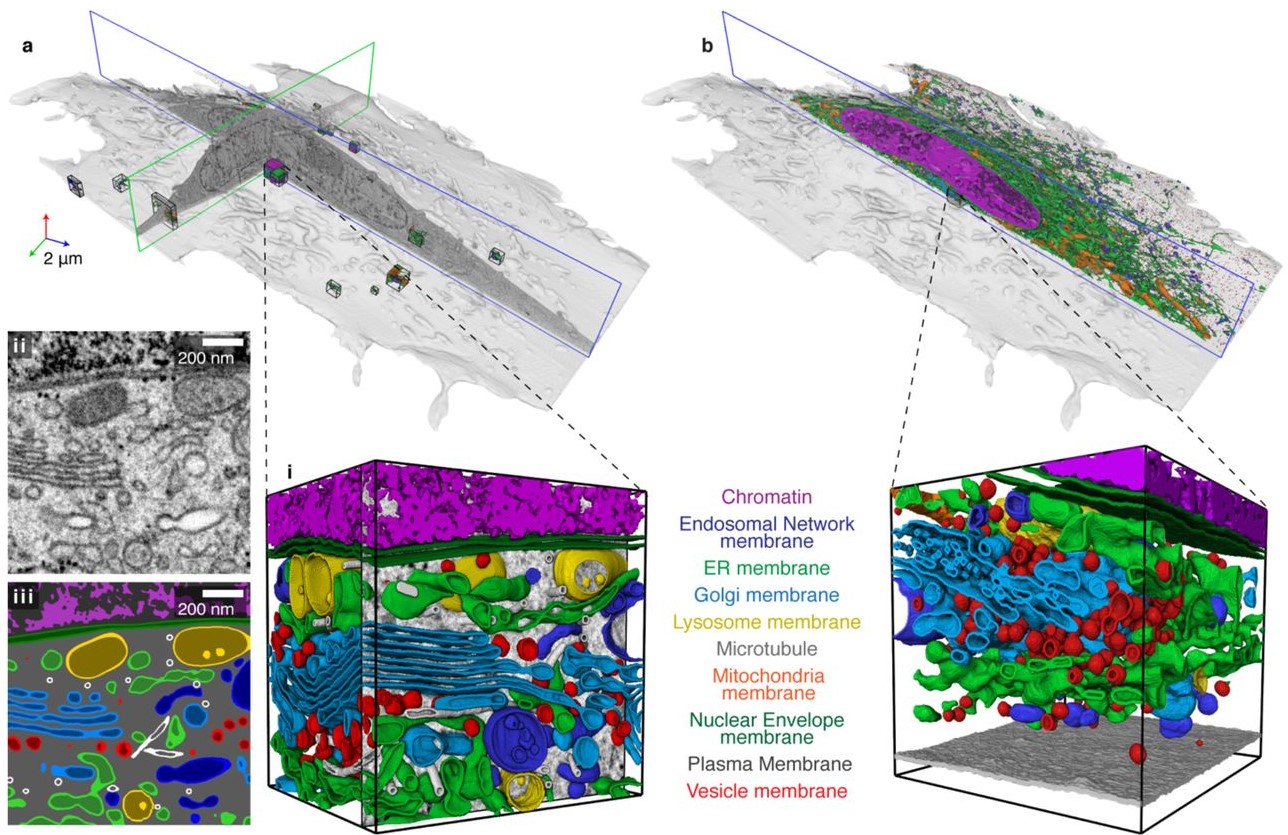
To meet this challenge, we developed an analysis pipeline for comprehensively reconstructing and analyzing the cellular organelles in entire cells imaged by focused ion beam scanning electron microscopy (FIB-SEM) at a near-isotropic size of 4 or 8 nm per voxel. The pipeline involved deep learning architectures trained on diverse samples for automatic reconstruction of 35 different cellular organelle classes – ranging from endoplasmic reticulum to microtubules to ribosomes – from multiple cell types.
Automatic reconstructions were used to directly quantify various previously inaccessible metrics about these structures, including their spatial interactions. We show that automatic organelle reconstructions can also be used to automatically register light and electron microscopy images for correlative studies. We created an open data and open source web repository, OpenOrganelle, to share the data, computer code, and trained models, enabling scientists everywhere to query and further reconstruct the datasets.